Architecting with Google Compute Engine - Design Process ch2. Business Logic Layer
Business_logic Layer
Design OverView
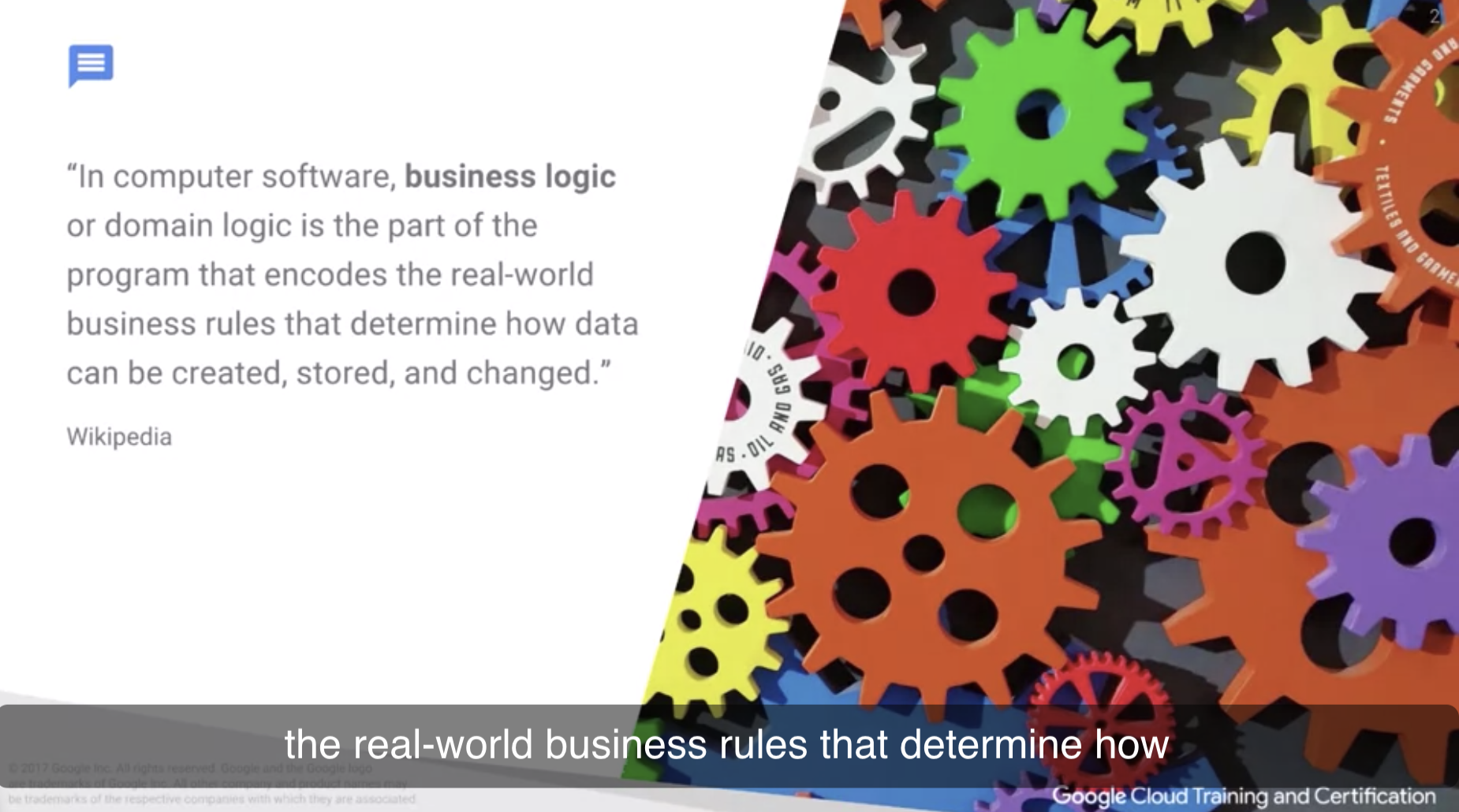
Business Logic = code that implements business logic (Computer Science에서의 정의) that determines what happens to data. = processing.
Ex) 비행기 티켓 예매라고 하면, 예매 인터페이스가 앱이건 키오스크건 상관없이 ‘티켓 예매’라는 데이터 처리는 동일하다. 이게 business logic.
- Microservices = specific kind of Service Oriented Architecture (SOA) -> leverages small, stateless processing for improving scalability and resiliency.
일반적으로 Application Design Approach에서 많이 사용됨.
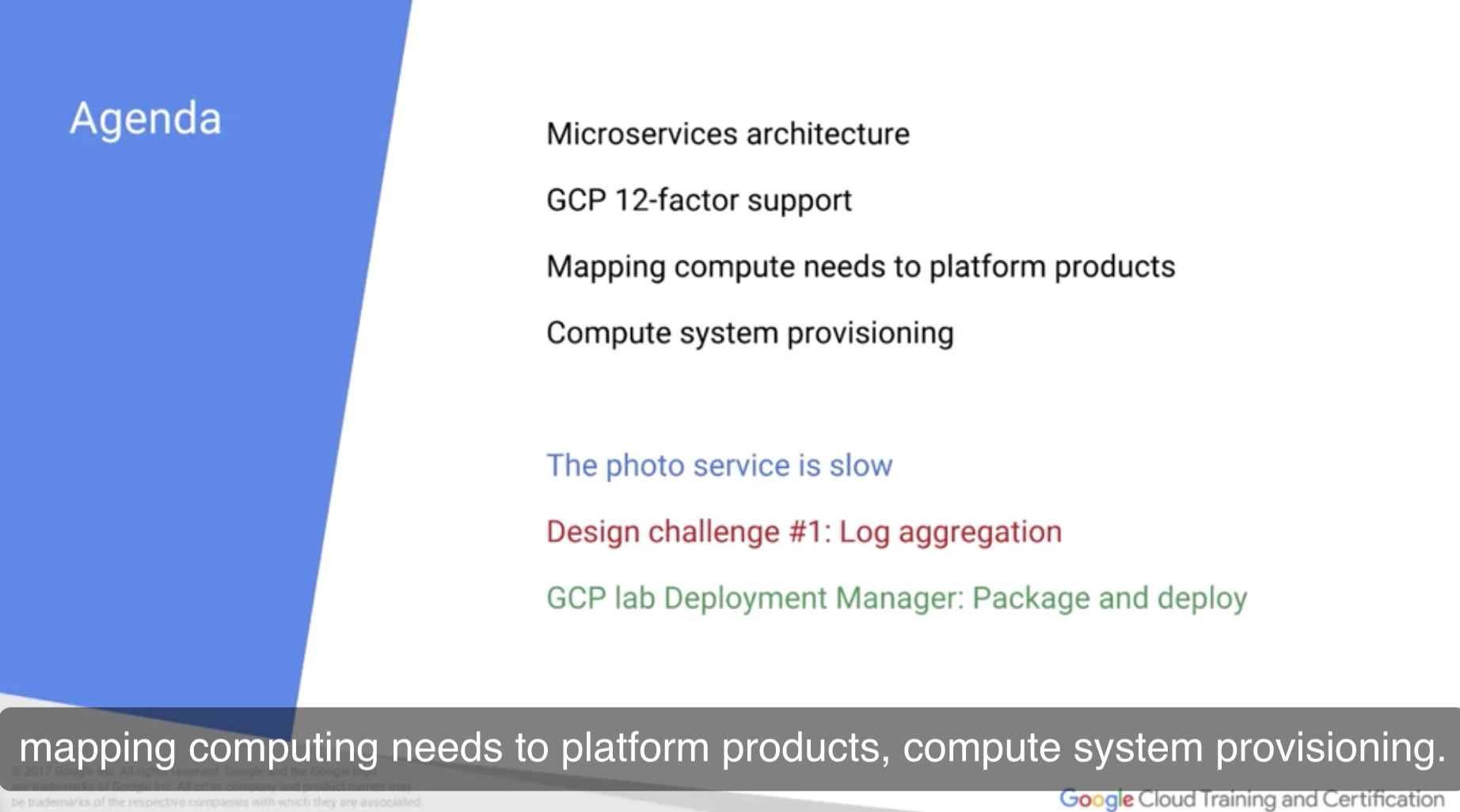
Business logic Layer : Microservice Architecture
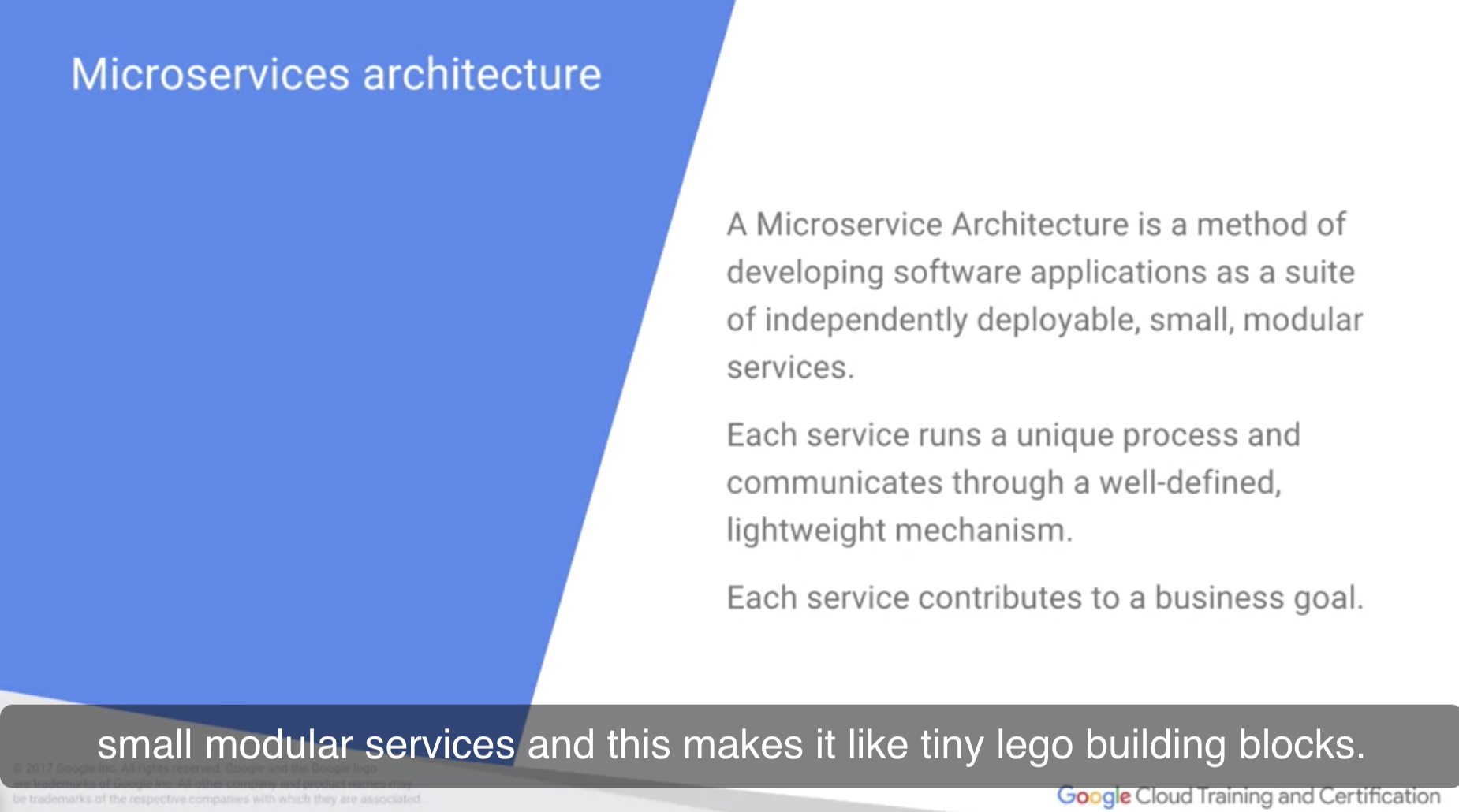
- Small modular Services that independently deployable을 결합하는 식의 Developing SW application 방법론. (Tiny lego block) .
- 각각의 service는 runs as a unique process, communicates through well-defined lightweight mechanism.
Ex) pub/sub. 메시지나 payload 보내고 받는 작업 자체가 lightweight mechanism - 각각의 서비스 자체가 individual goal이 존재함. 너무 micro하면 안 됨
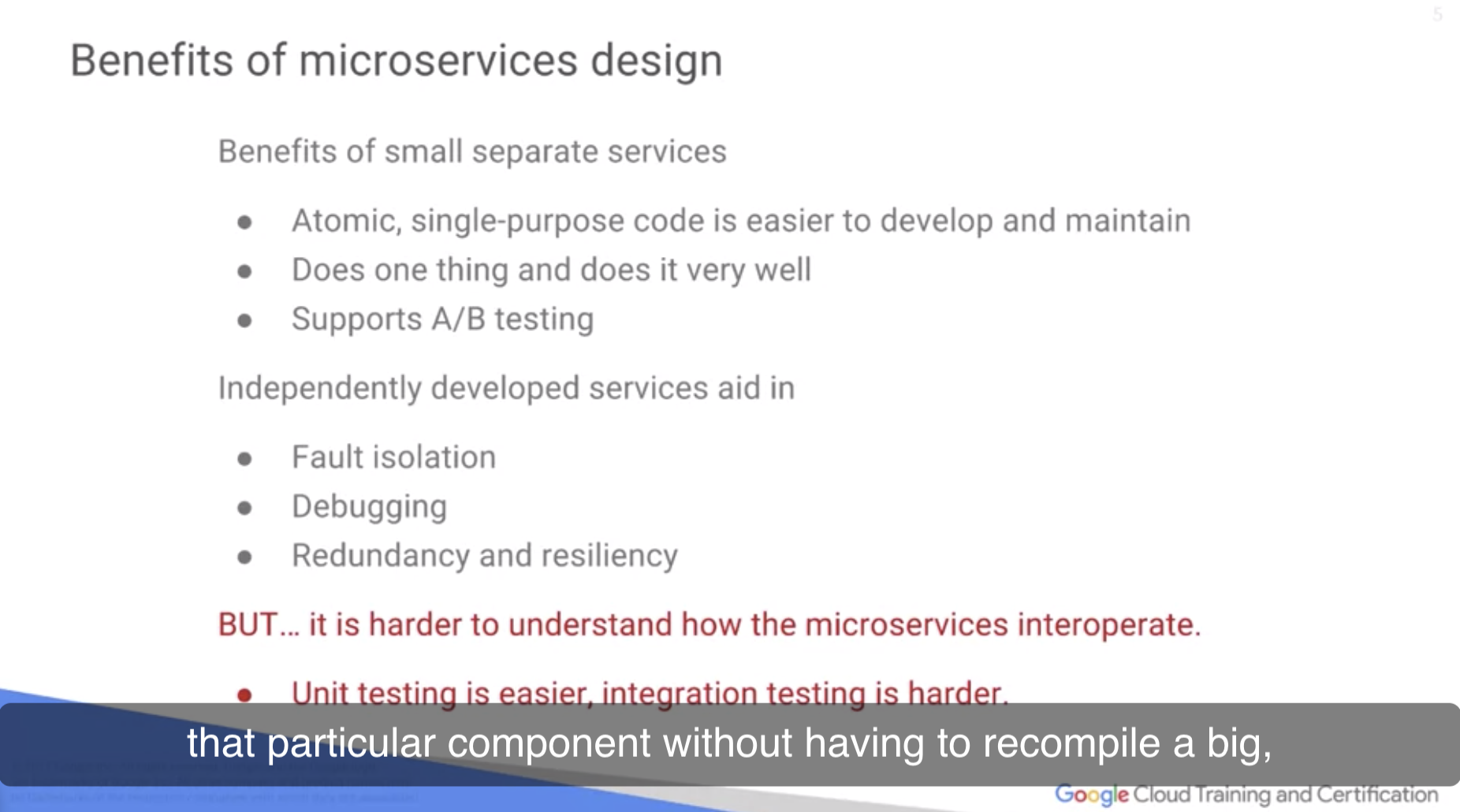
쓰는 이유?
- Atomic. Single-purpose code which makes it very easy to develop and maintain. 따라서 troubleshooting이 쉽고 빠르다. 각각의 함수마다 목적이 명확히 있기 때문에 필요하면 갖다쓰는 식으로도 적용 가능 (API처럼)
- AB Testing 지원. 특정 component만 갈아끼우는 식으로 변경할 수 있기 때문에, 프로그램 전체를 re-compile하는 등의 작업이 필요 없음.
- Independently developed service -> fault isolation, debugging, redundancy and resiliency 지원. (Make clones as we need to satisfy our requirements)
단점
- Interoperate 과정을 이해하기 쉽지 않다. 모든 service는 not rely on each other, but 통신을 위한 규칙이나 매커니즘은 있어야 함.
- 1과 연계되는 문제로, unit testing은 쉬워지지만 integration testing이 복잡해진다. 어디서 발생한 문제가 어디에 영향을 미치는지 예측이 쉽지 않은 편
(Airline Service 같은 거. Weather delay / 항공기 지연도착 등의 문제… creates the huge chain of events. Airlines 자체가 Microservices at a very grand scale)
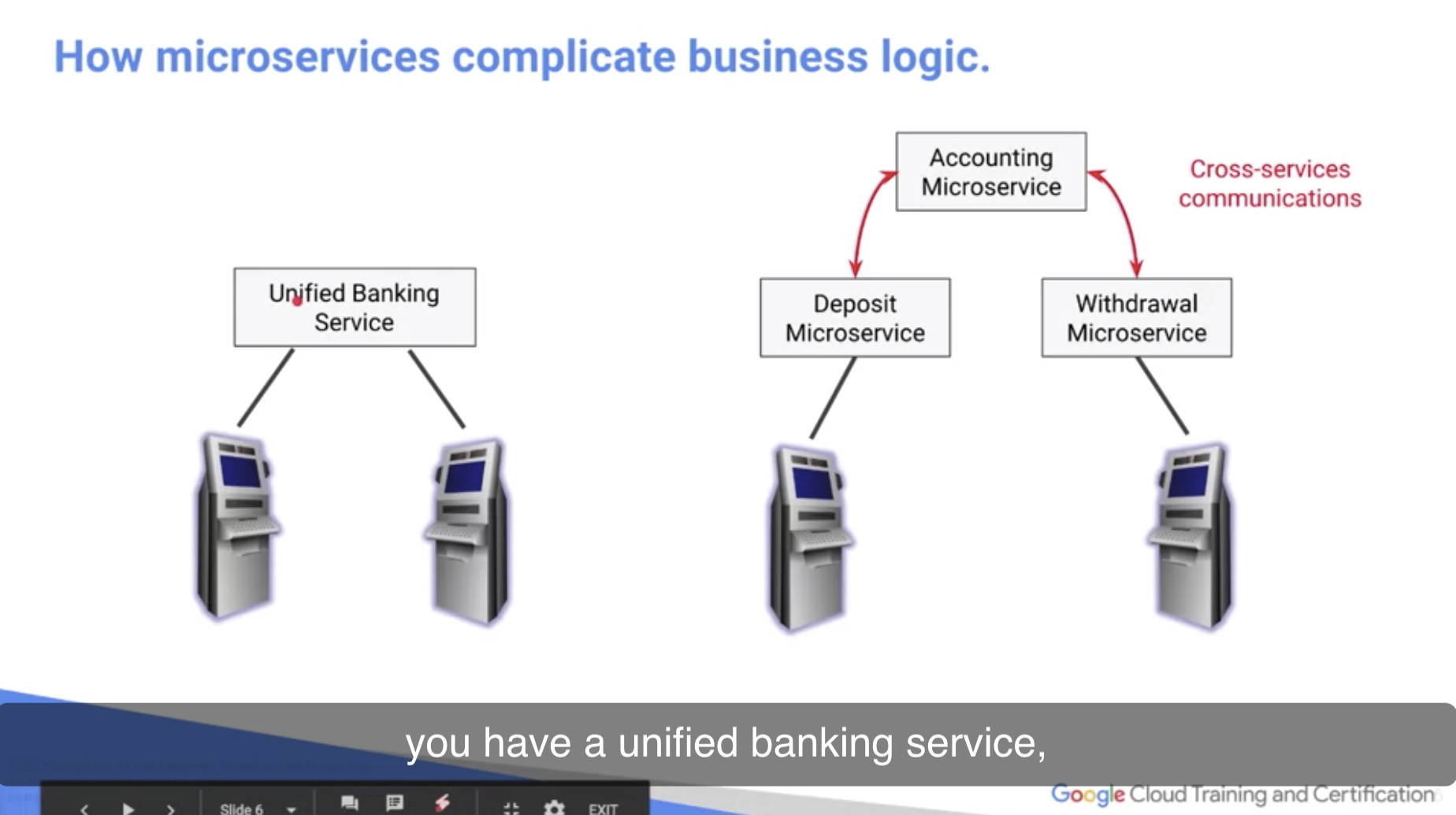
예시로 제시한 ‘은행의 송금 시스템’을 Microservices 형태로 구현할 경우. 누군가의 계좌에서 돈이 출금되는 것 / 누군가의 계좌로 돈이 입금되는 것. 이 두 가지가 independent Service가 된다. 단, 여기서 입출금은 atomicity가 보장되어야 하기 마련. atomicity를 만족할 수 있는 communication 방법론이 여기서는 필요한 셈이다.
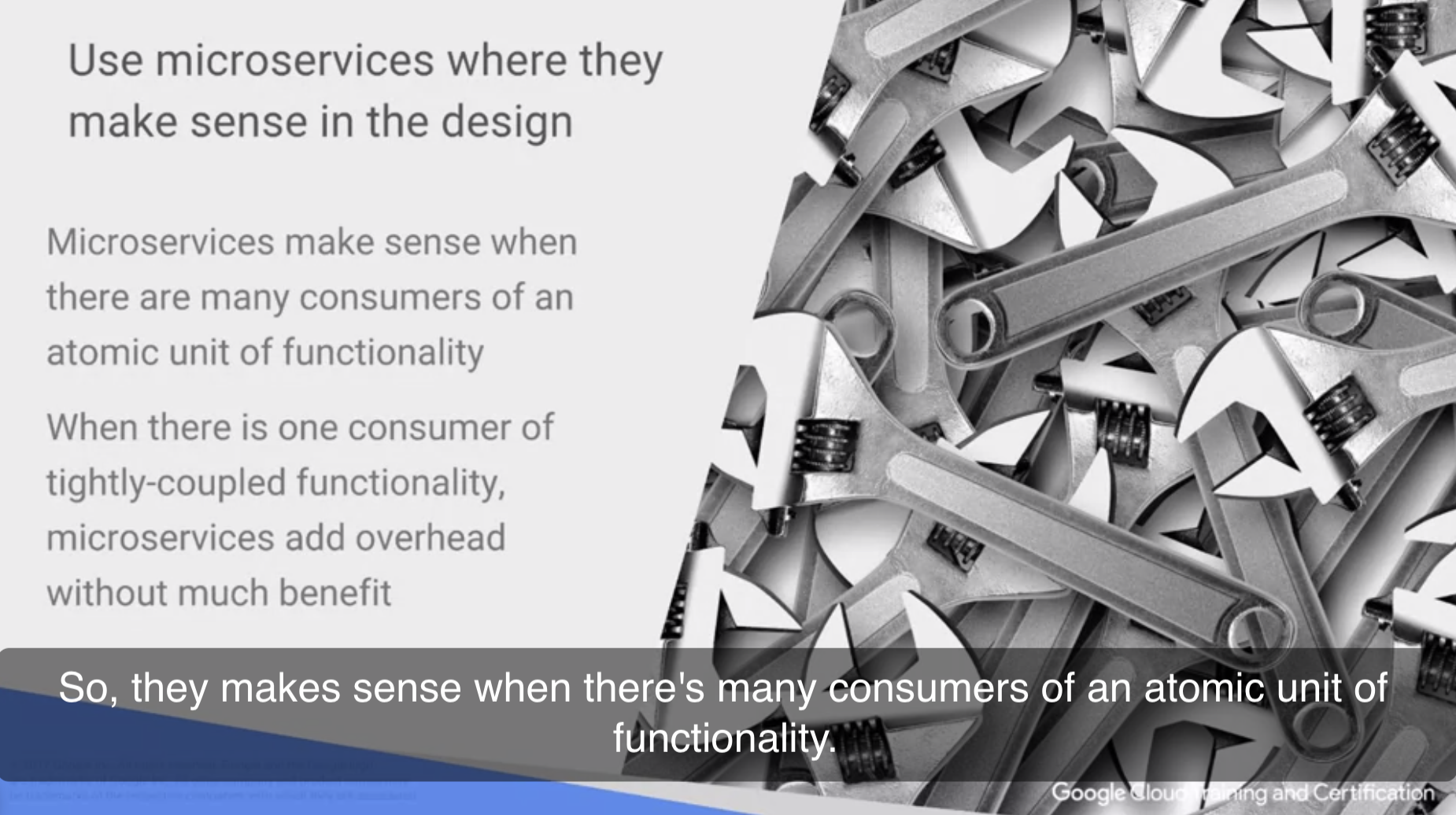
결국 요지는, ‘필요한 곳에 MSA 디자인을 활용할 줄 알아야 한다’.
Many customers of an atomic unit of functionality일 때. 다시말해 많은 사람들이 똑같은 걸 계속 반복할 때 적합하다.
이미지 처리 앱의 경우에도, 이미지 업로드 -> 처리 과정이 계속 반복된다면, 이미지 업로드 함수 따로 / 처리 함수 따로 작업하는 게 맞다는 것.반대로 말하면, one consumer of tightly coupled functionality일 경우에는 MSA는 별 도움이 안 된다. Tightly coupled functionality를 encapsulate intro one consolidated service -> 더 효율적이기 때문.
MSA를 구글이 지원하는 방식
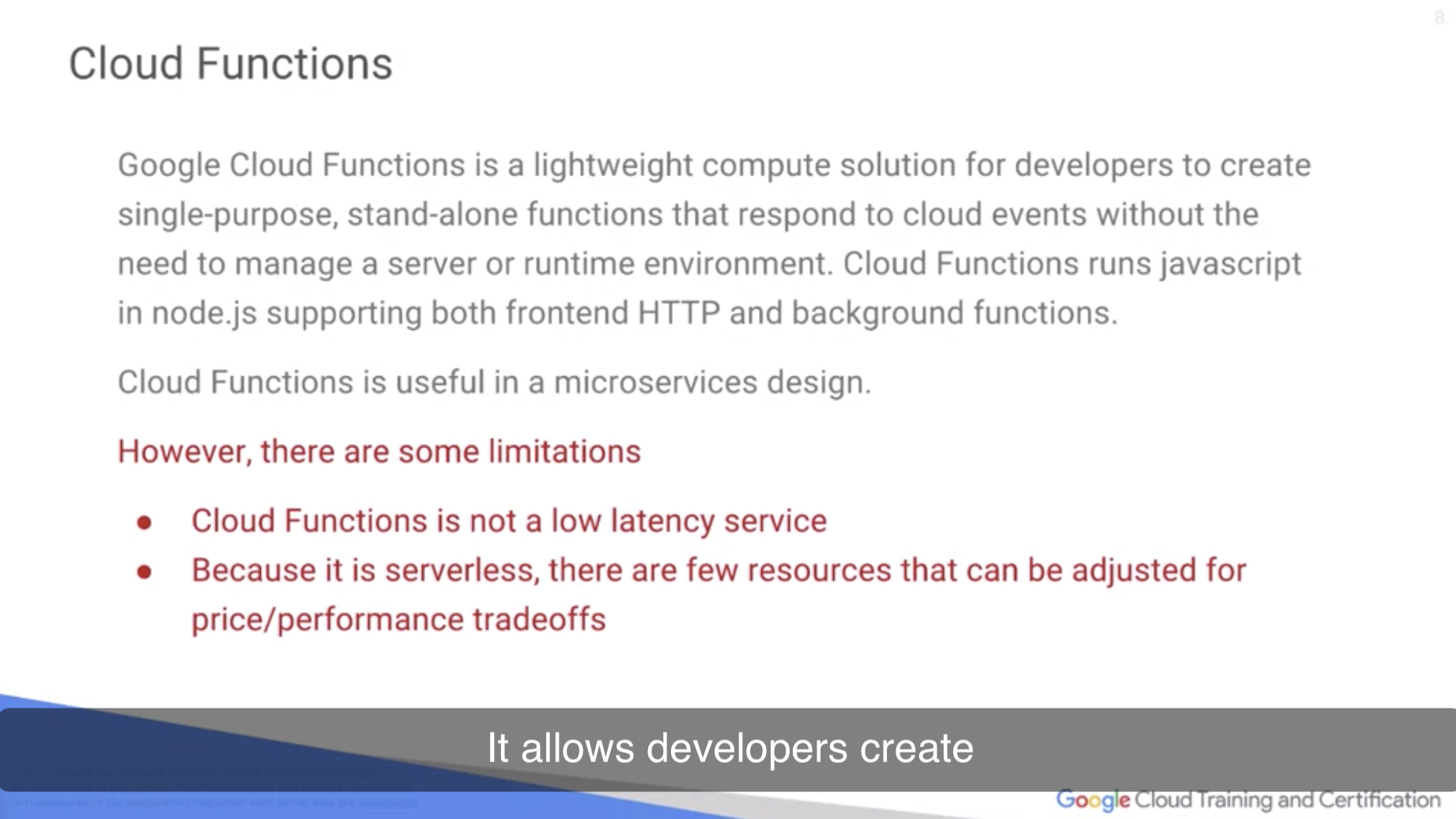
- Cloud Function : Managed Service로, lightweight computes solution that allows developers create the single-purpose standalone functions that respond to Cloud Events.
JS on Nodejs 형태. 따라서 frontend / backend function 전부 지원한다. MSA 디자인에 가장 적합한 기능. 자기 업무가 다 끝난 뒤에는 다른 serivce에 make call하는 것도 가능하다.
유의점: not intended for low latency. latency가 있다는 거 감안하고 써야 함. Scale 작업하면서 latency가 중요해질 경우 다른 서비스를 써야 한다. Kubernetes라던가, 아니면 customized Compute Engine이라던가.
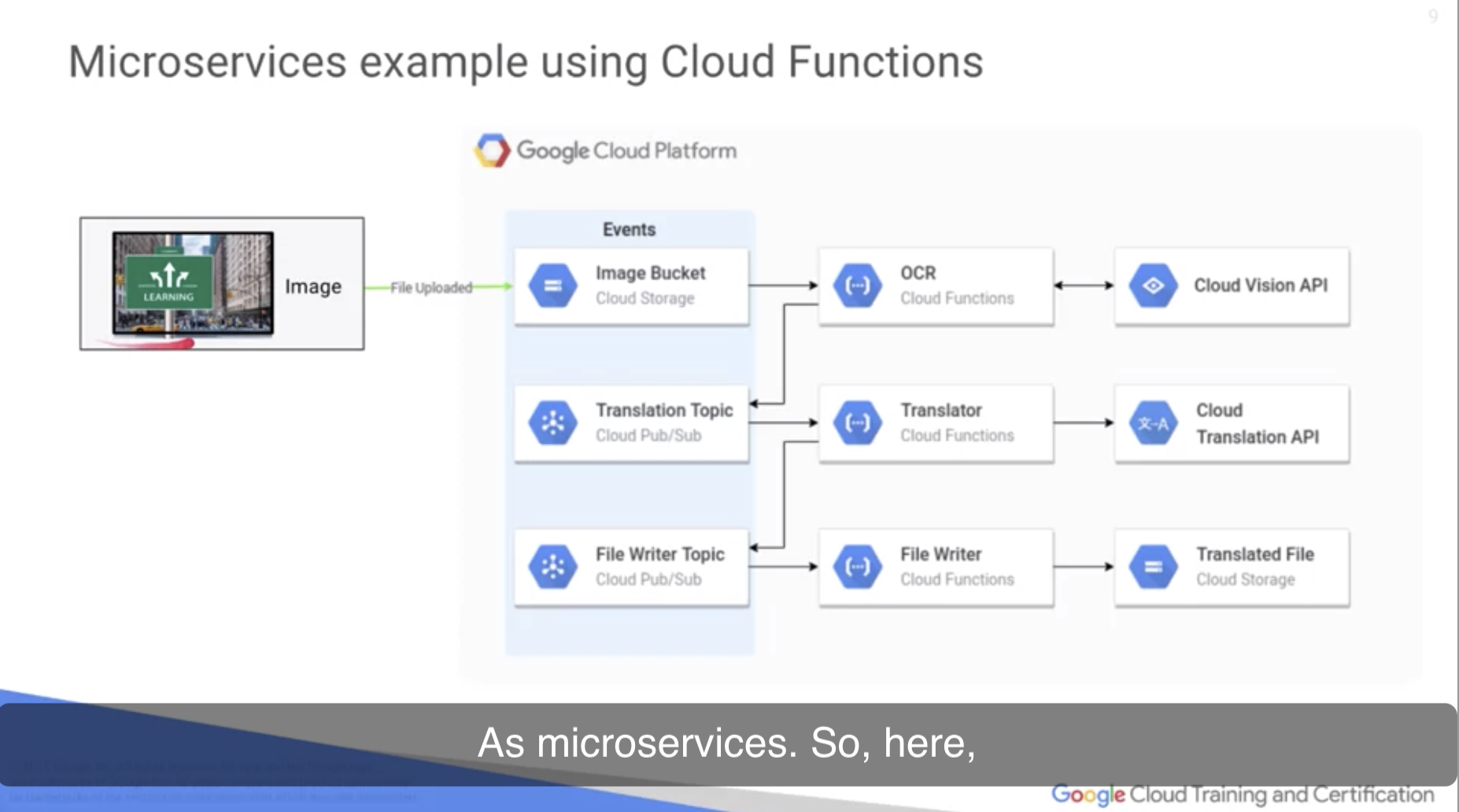
강의의 예시를 microservice로 분할한 모습
Google App Engine으로 MSA 디자인 작업하기
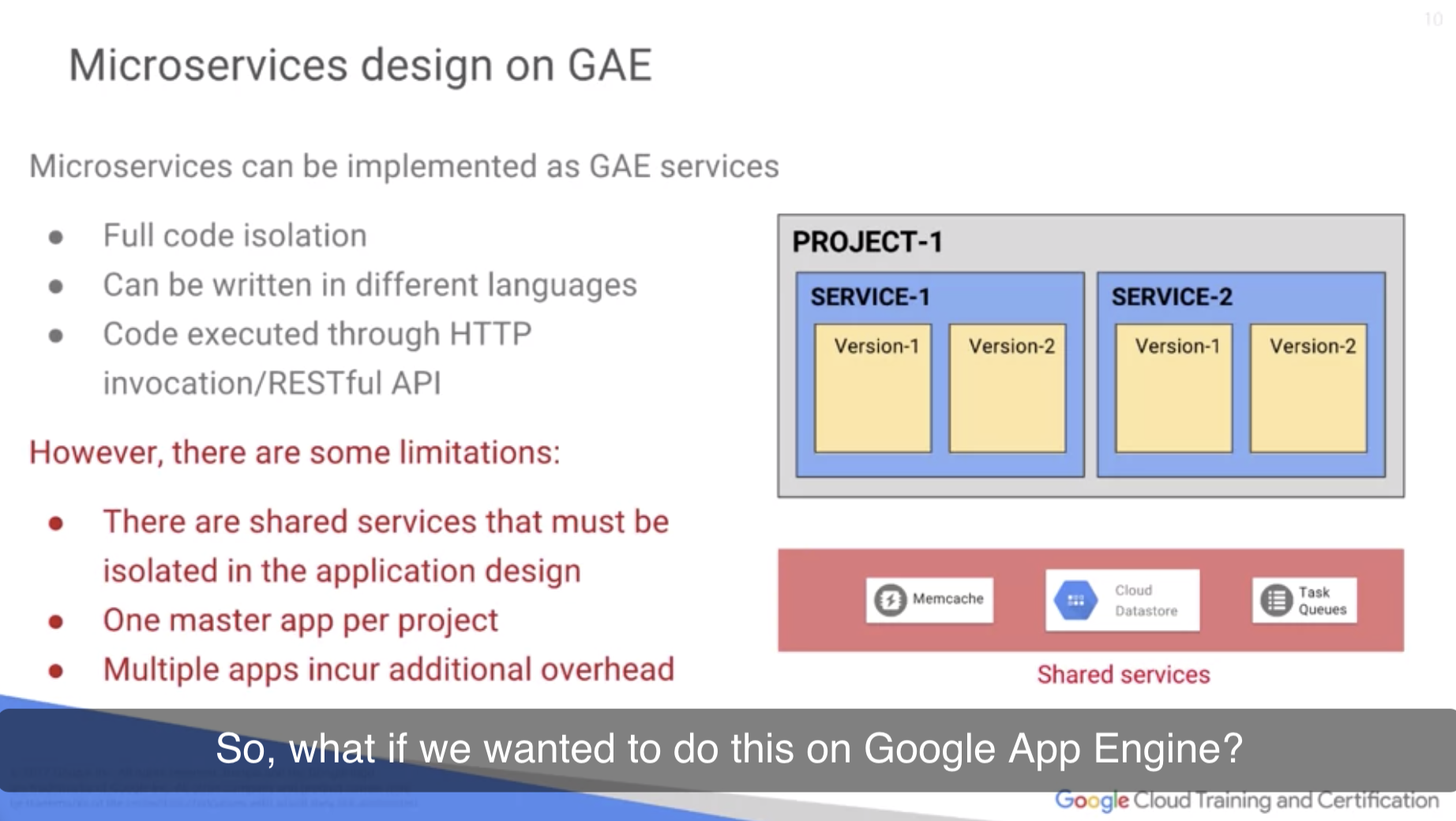
App Engine 사용 시
- 장점: logic code에만 집중할 수 있다. 언어제약 없음. http나 restful api 사용 가능
- 단점: one master application running in production per project. Local File System 접근 불가능. state를 저장하기 위해서는 external service를 사용해야 한다.
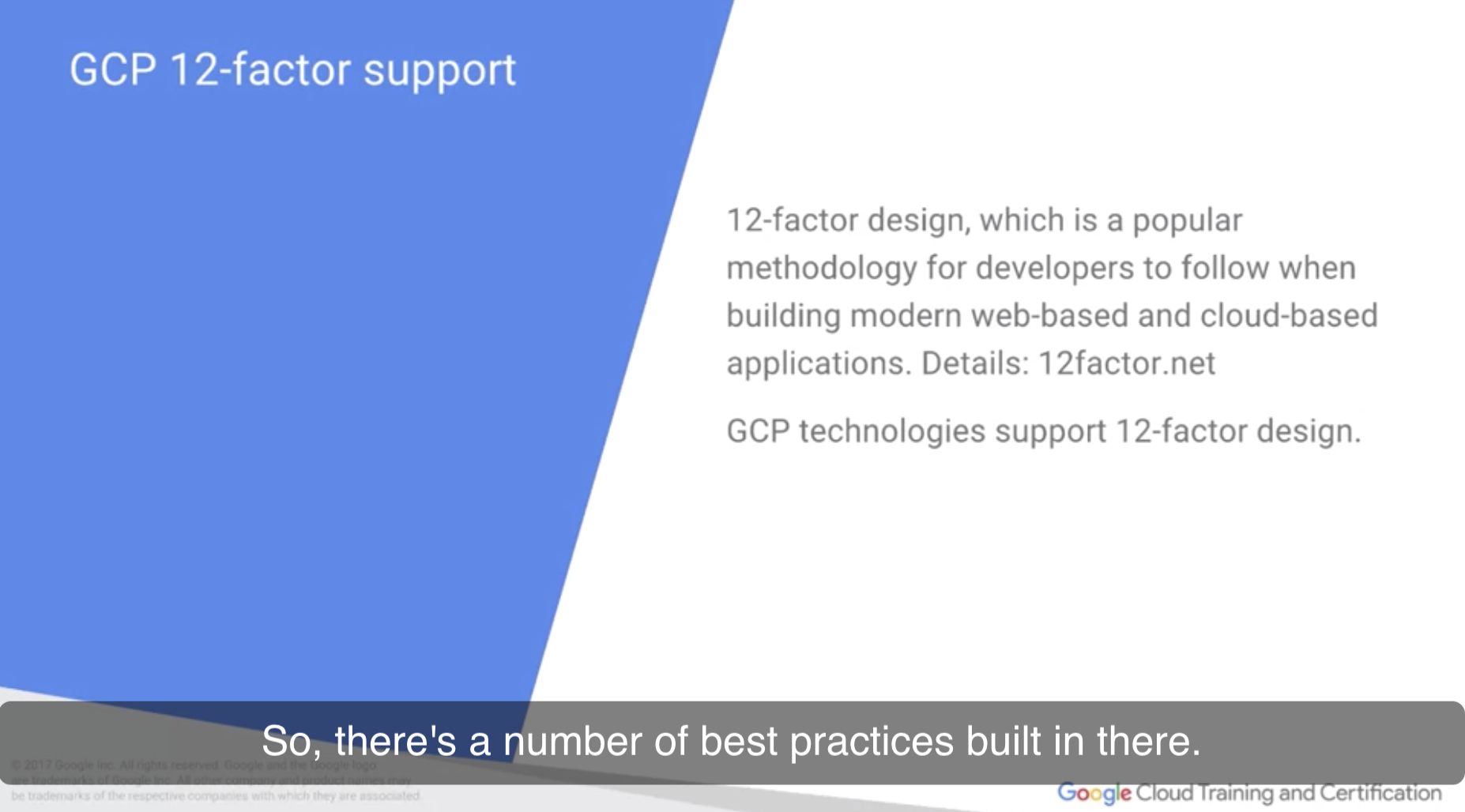
Web based, cloud based Application을 개발할 때 적용 가능한 12개 factor design.
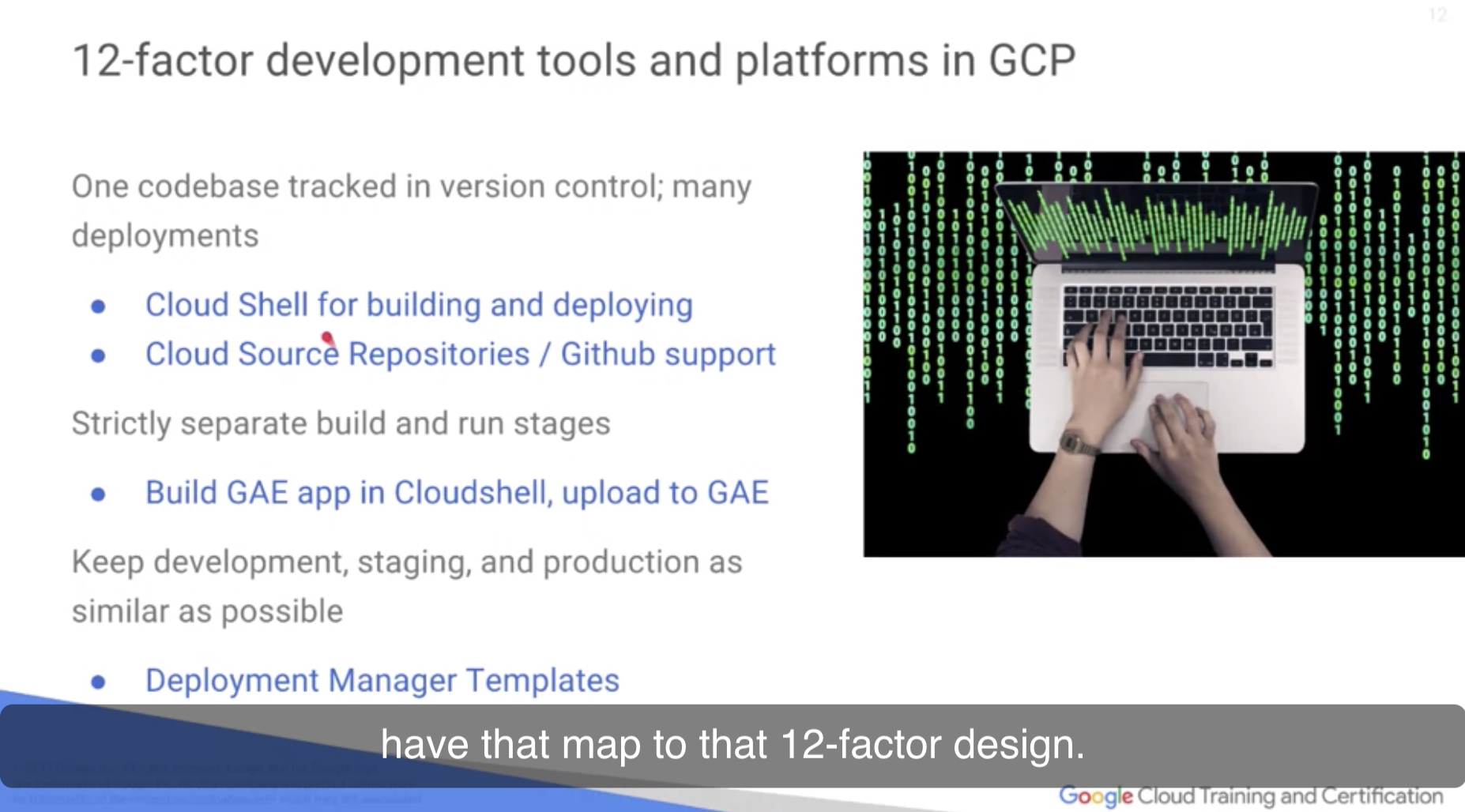
Single Codebase tracking in version control. 이렇게 하면 multiple / many different deployment가 가능하다.
- Cloud Shell 활용한 개발. Baston host이며, 엥간한 API 호출에 우선순위가 있음. Micro VM이며, storage 5GB 정도. API developer tool 지원하며 자동으로 업데이트.
- Cloud Source repository. Private github 느낌. 깃허브와 연동도 된다
Strict separating build, run stage
- App Engine 활용하기
Dev staging과 production staging 일치시키고 싶을 경우
- Automation = Deployment Manger Template.
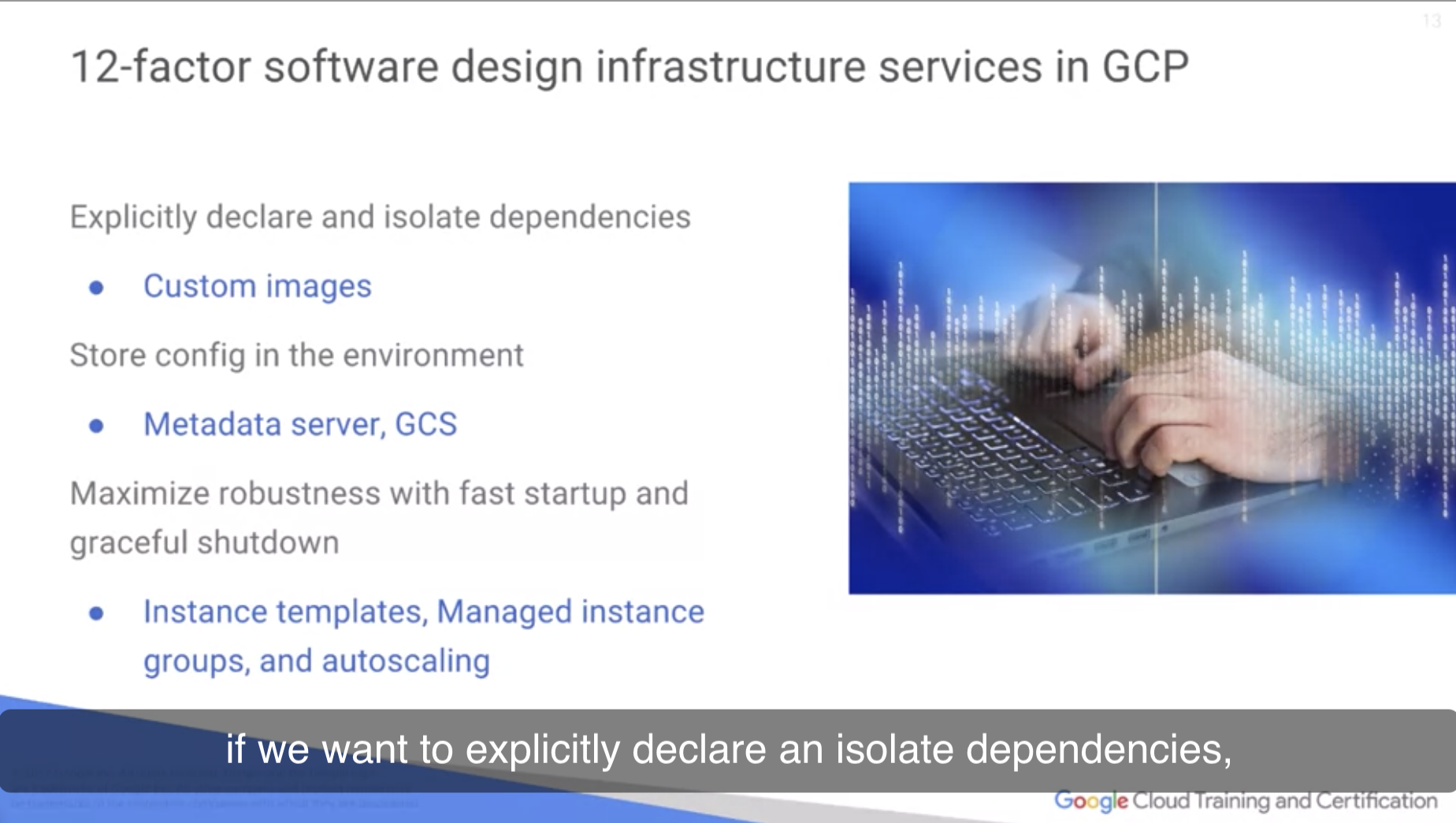
Custom Image : own separated source code repository with different versions control.
Store config in the environment : 보통 on your own으로 처리하려면 골치아픈 지점. External dependency가 다들 있기 마련이니까. Github에 개인 비밀번호나 중요한 환경변수를 올릴 수는 없듯.
따라서 metadata server에 해당 정보를 올리고, Google Cloud Storage에서 called externally 할 수 있게 설정하는 식.
Startup Script에 pull off dynamic attributes where your code can communicate with some Services. (코드에서 “로직은 알고 있으니, 필요한 개발환경이나 external ip, authentication token 등을 제공해달라”고 요구하는 식)
- Managed instance groups -> dynamically scale up / down, use same configurations, auto-scaling.
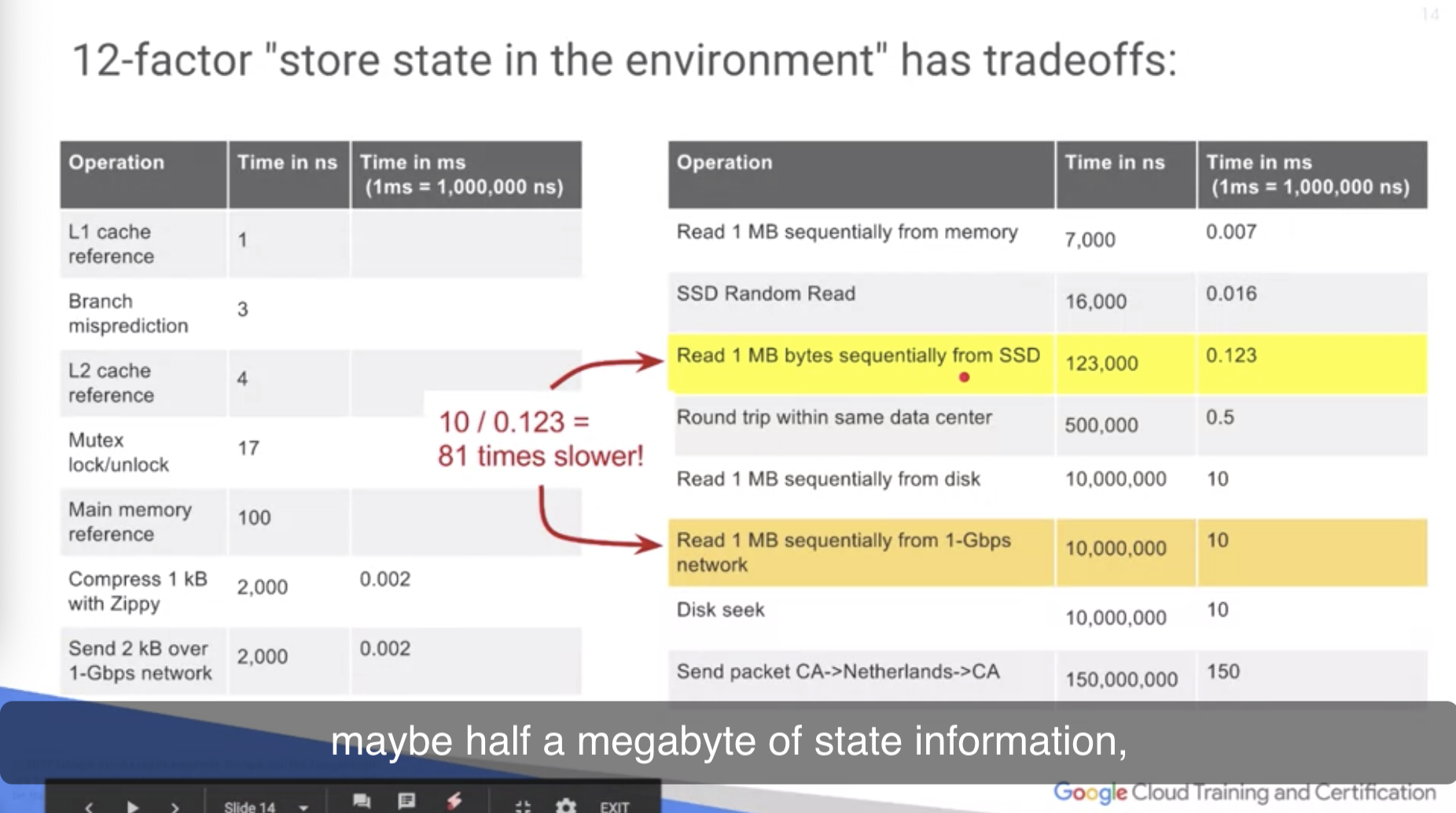
Storing state in metadata는 좋은 방법이지만, 만약 local HW에 저장하려면?
- SSD에 저장할 경우, 뭘 저장하든 상관없이 low latency는 보장한다. 대신 scale 할 경우에는 not recommended (concerns with distributing your state)
- Cloud Storage나 NFS mount 사용 시 -> dynamically increase latency dramatically.
즉, 단순히 offload the state -> 디자인 과정에서 고려할 사항 맞다.
How dependent where you on those performance도 고려해야 할 요소 중 하나.
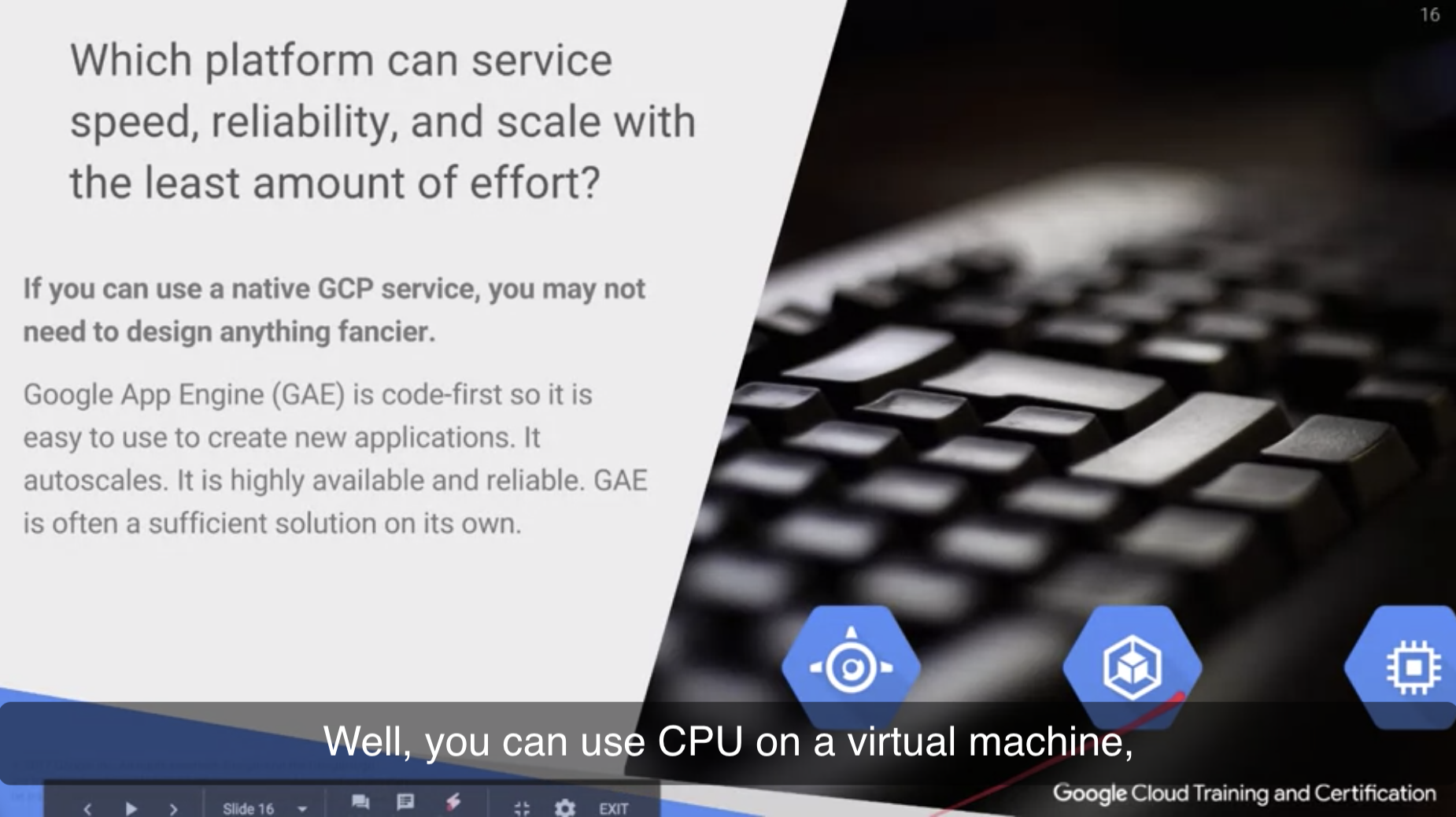
Business logic인 Application을 굴릴 경우, resource를 쓰게 된다. 어떤 resource가 가장 적합한가?? 의 문제. 필요한 서비스를 어떤 식으로 제공받는지에 따라 선택할 Compute Engine 서비스가 달라질 거다.
- App Engine = get up and running이 매우 빠름. Environment 수정할 필요 없고, 그냥 ramification (파급효과) 만 인지하고 있으면 된다.
- 더 디테일한 설정이나 정리가 필요하면 Containerization (근데 요즘은 App Engine에서도 컨테이너화 가능하다고 함)
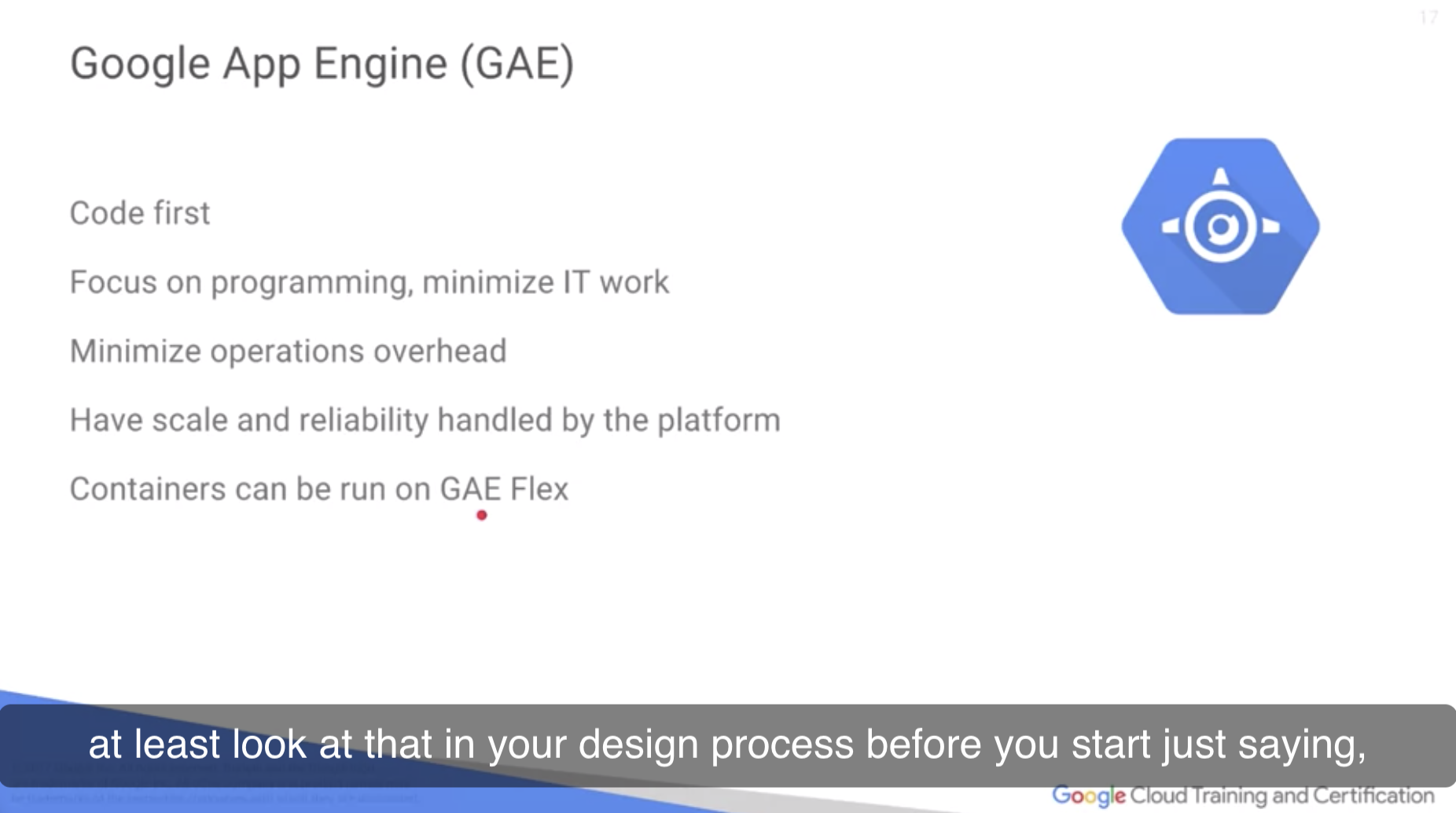
GAE. 장점은 여러 번 상술했으니 건너뛰고, GAE Flex가 Container 지원하는 App Engine 서비스라고 보면 된다. 아무튼 처음 작업할 거라면 얘부터 한번 고민해 보는 걸 추천.
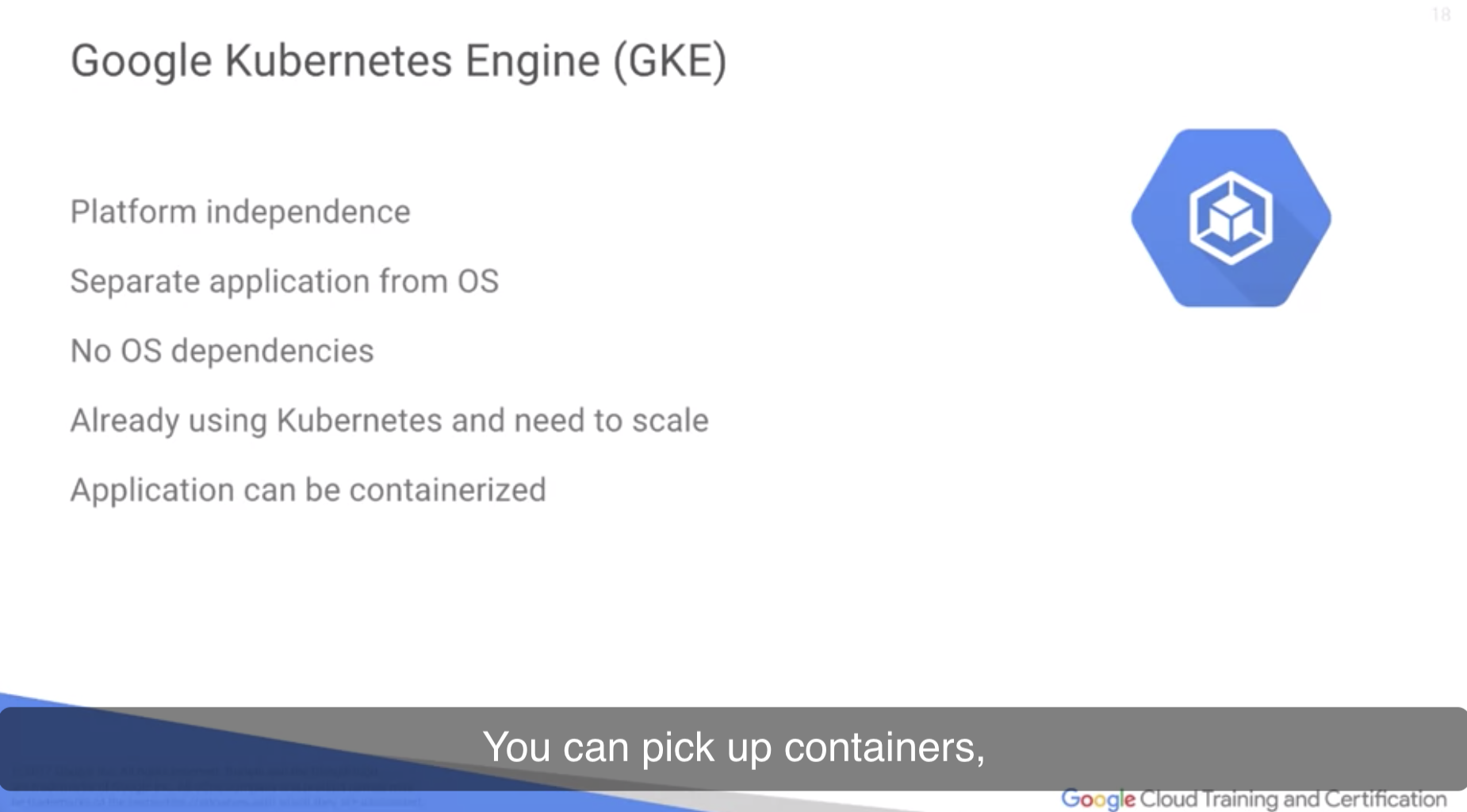
Container 기반 Kubernetes. Complete platform independence가 제일 큰 장점. App Engine을 사용할 경우, 구글에서 제공하는 API 위주로 작동하고 돌아간다. Upscale이라는 파트너사에서 clone App Engine -> allow you to do additional things (running in china 같은).
얘는 그런 거 없음. Flexibility 제공, scale 용이하고, kubernetes 이미 쓰고 있으면 그대로 옮겨올 수도 있다.
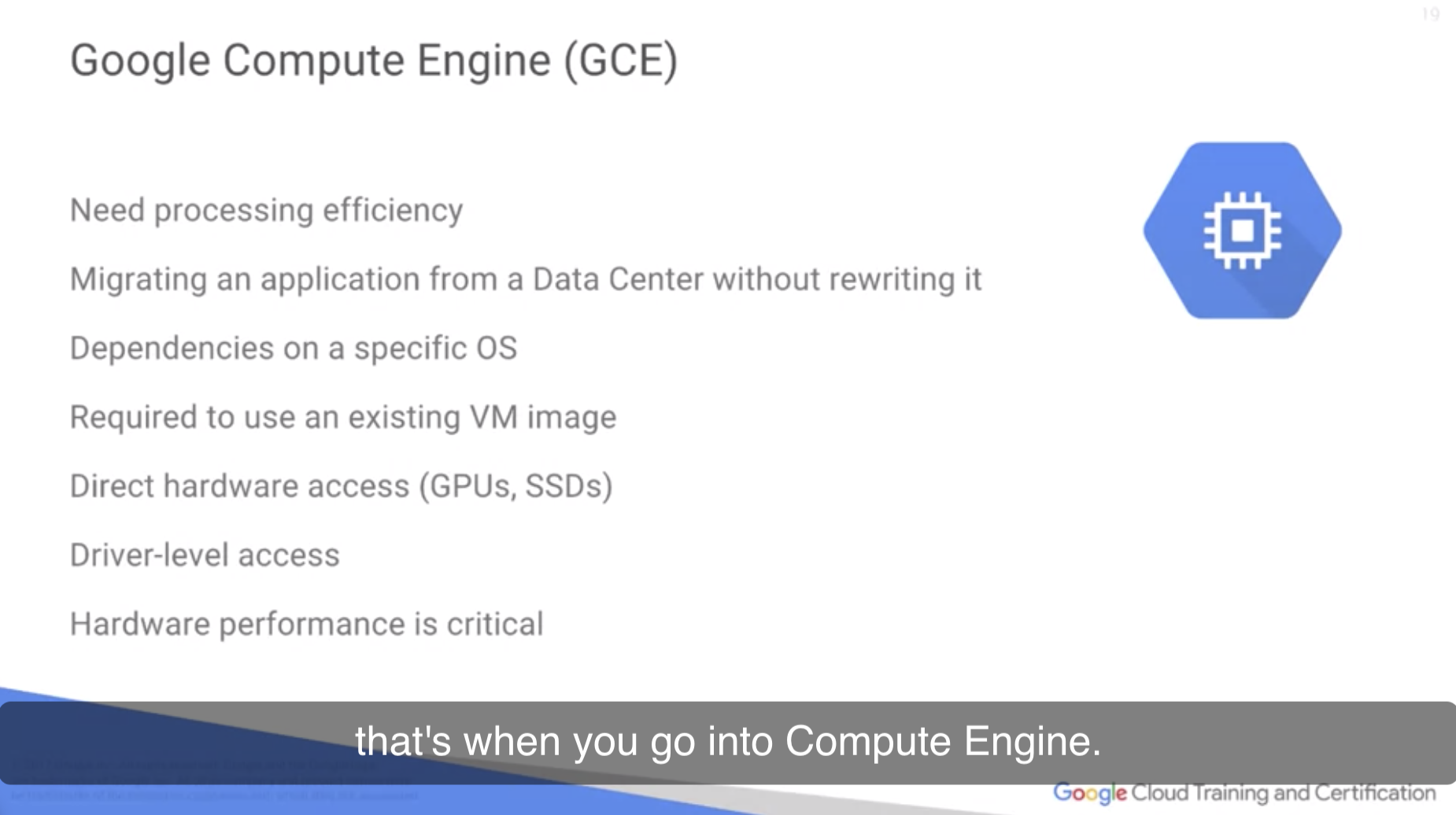
- Processing efficiency가 최우선일 경우 (HPC running = exact HW가 필요한 경우)
- Local Access가 필요한 경우 (SSD, GPUs, 특정 드라이버나 HW가 필요한 경우라던가)
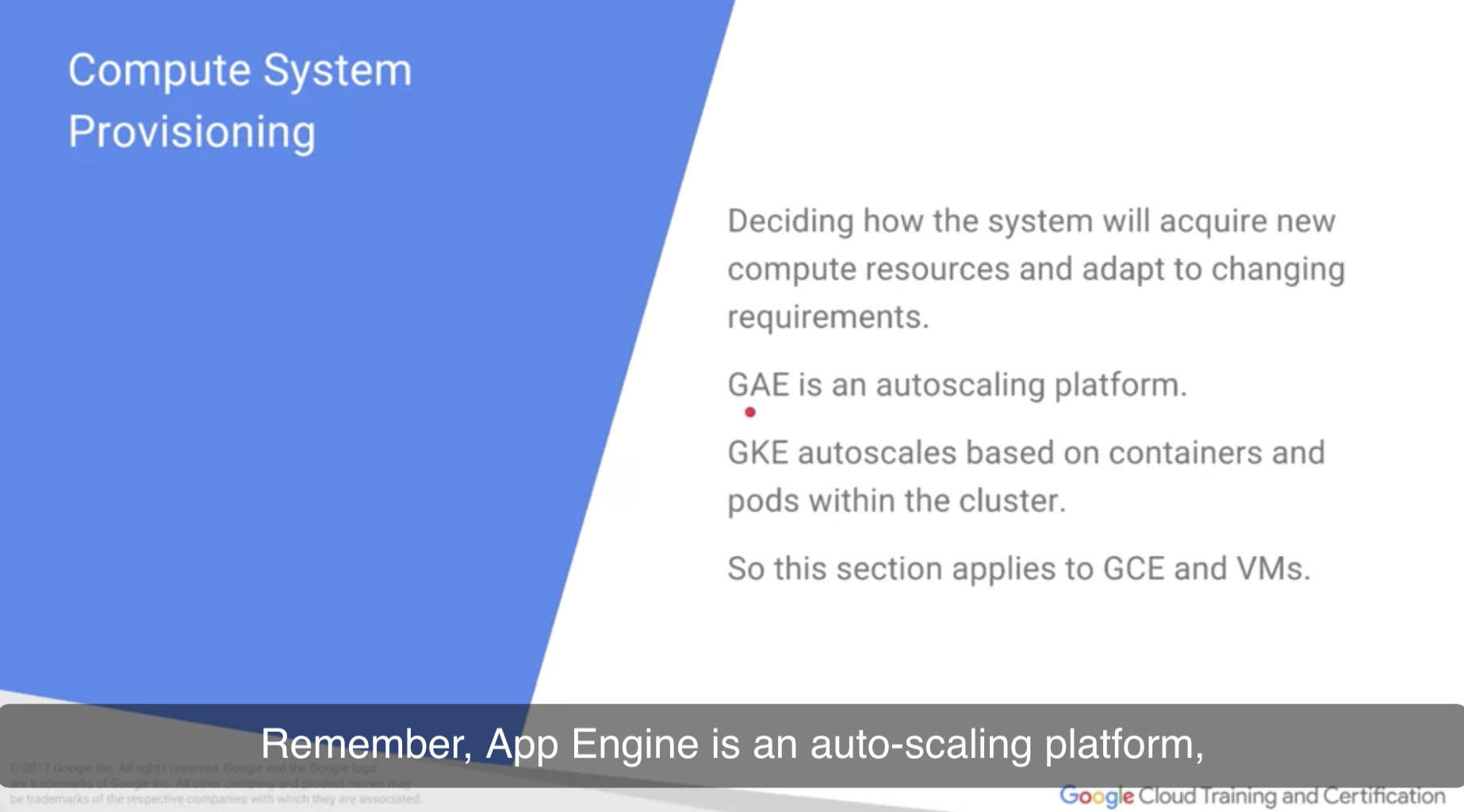
- App Engine : AutoScale
- Container Engine : AutoScale based on containers and pods within cluster
Compute Engine의 경우?
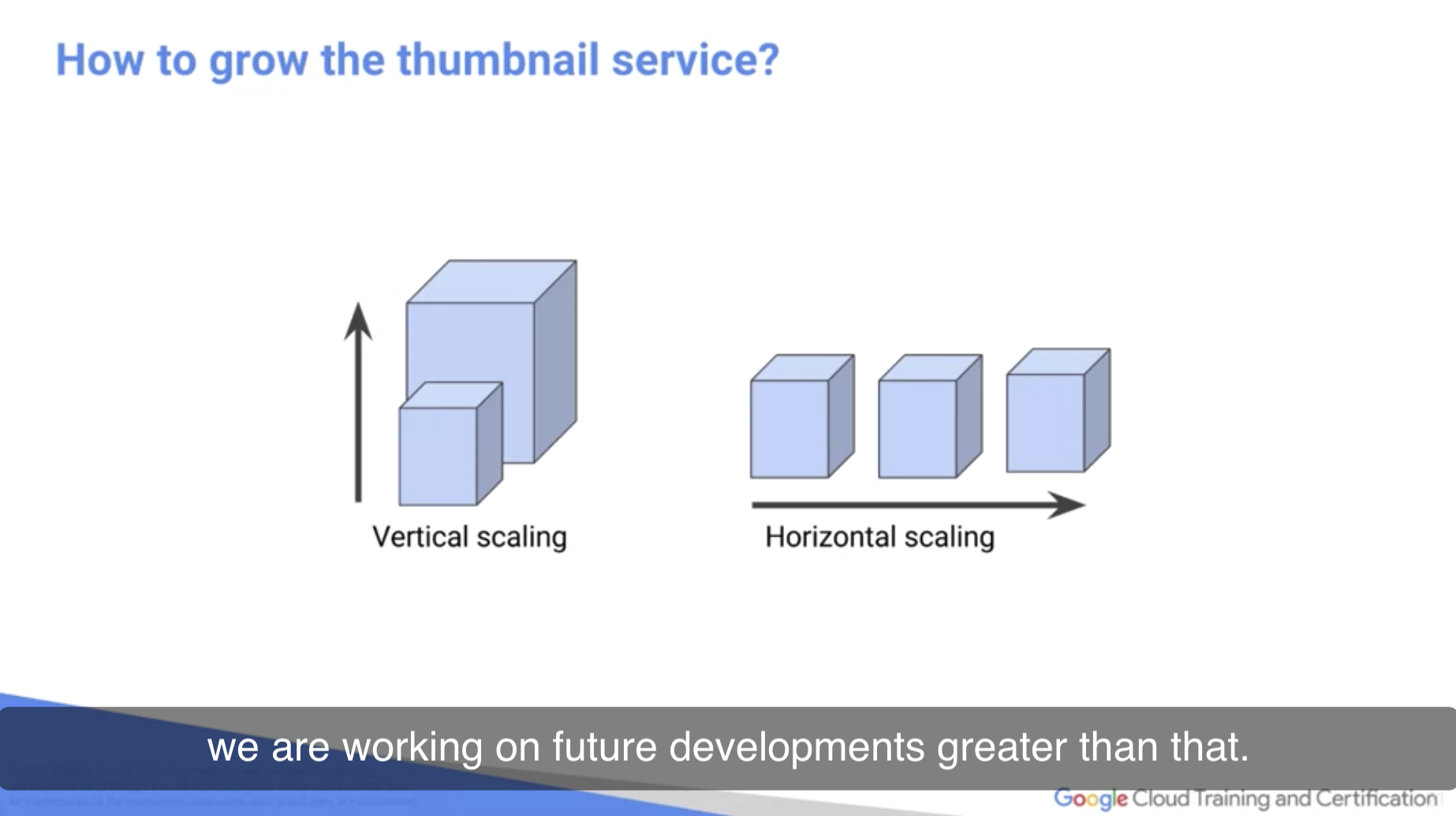
일반적으로 horizontal Scale이 많은 issue를 해결하는 좋은 방법임. 개발 초기 단계에서부터 horiziontal scale 고민하는 걸 추천한다.
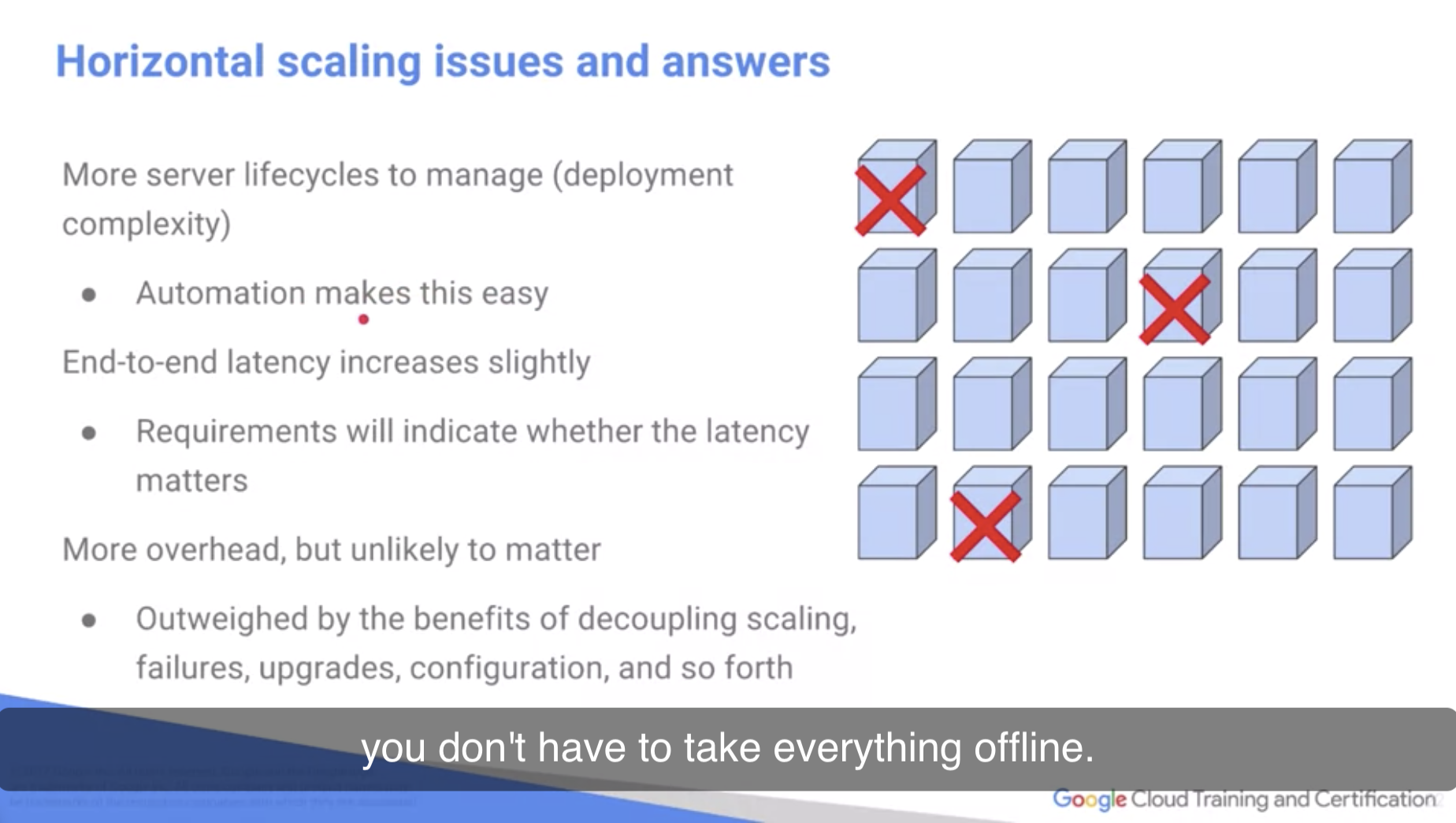
그렇다고 단점이 없는 건 아니고
- End-to-end latency가 증가한다. (단점이긴 한데, 다른 대안이 가진 단점 크기에 비하면 뭐)
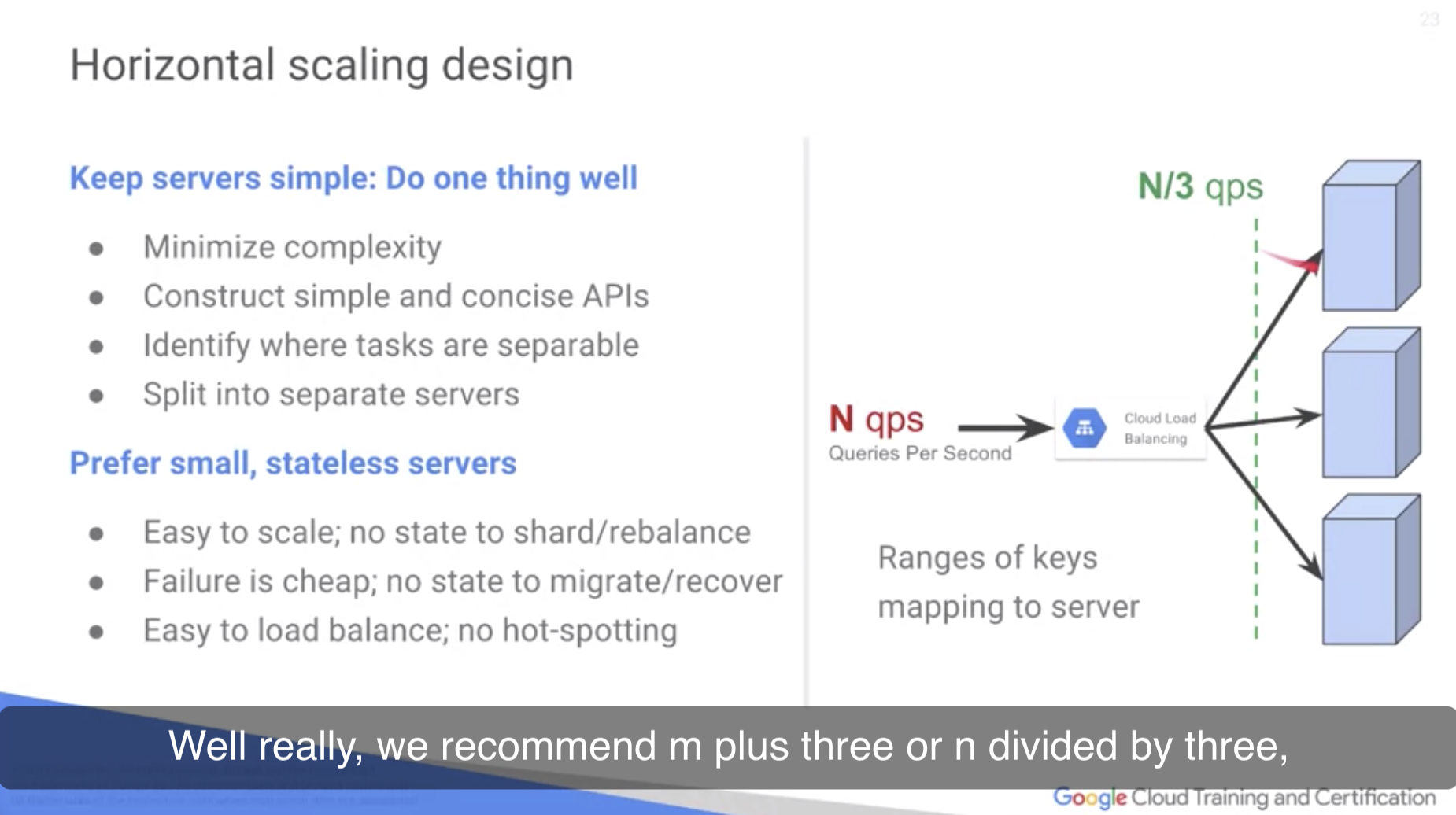
권장하는 양은 n+3이거나ㅏ, n//3 정도. Fault tolerance를 안정적으로 보장할 수 있기 때문.
- Goal : prefer small statelesss servers.
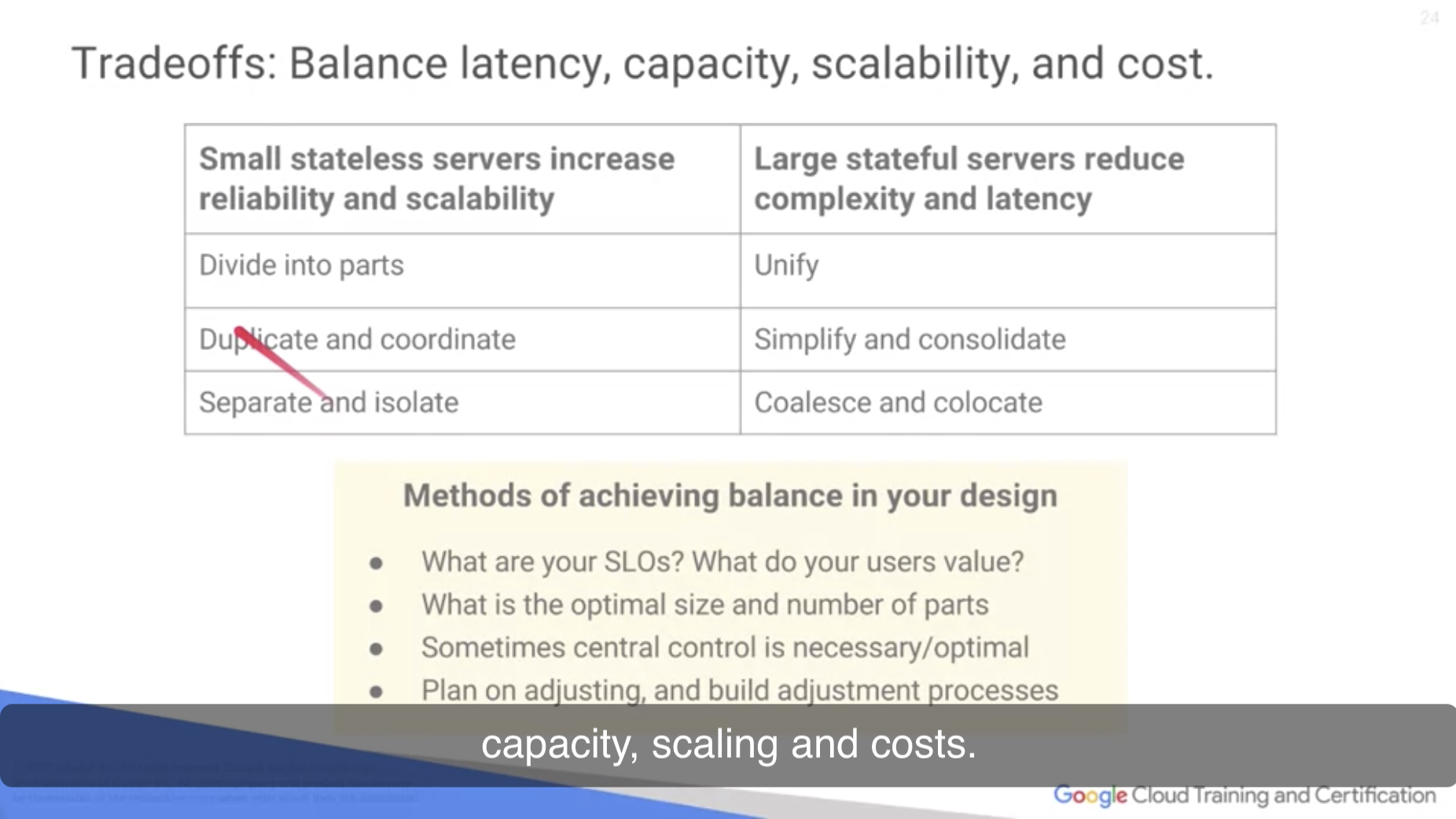
(처음부터 small stateless server 형태로 디자인하기 vs 큰 서버 하나 두기)
-> SLO 생각해라. 사용자에게 뭐가 더 중요한가?
Plan on adjusting도 중요하다. 서비스가 성장할 때, 모든 것이 linear grow하는 건 아니기 때문. Potential bottleneck 부분이 존재하기 마련이다. 그걸 미리 확인해보려고 benchmark / test scale을 진행하는 것.
-> bottleneck이 어디서 발생하는지 봐야 한다. 이게 App level인지, cloud level인지. 이걸 토대로 estimation을 해보는 것
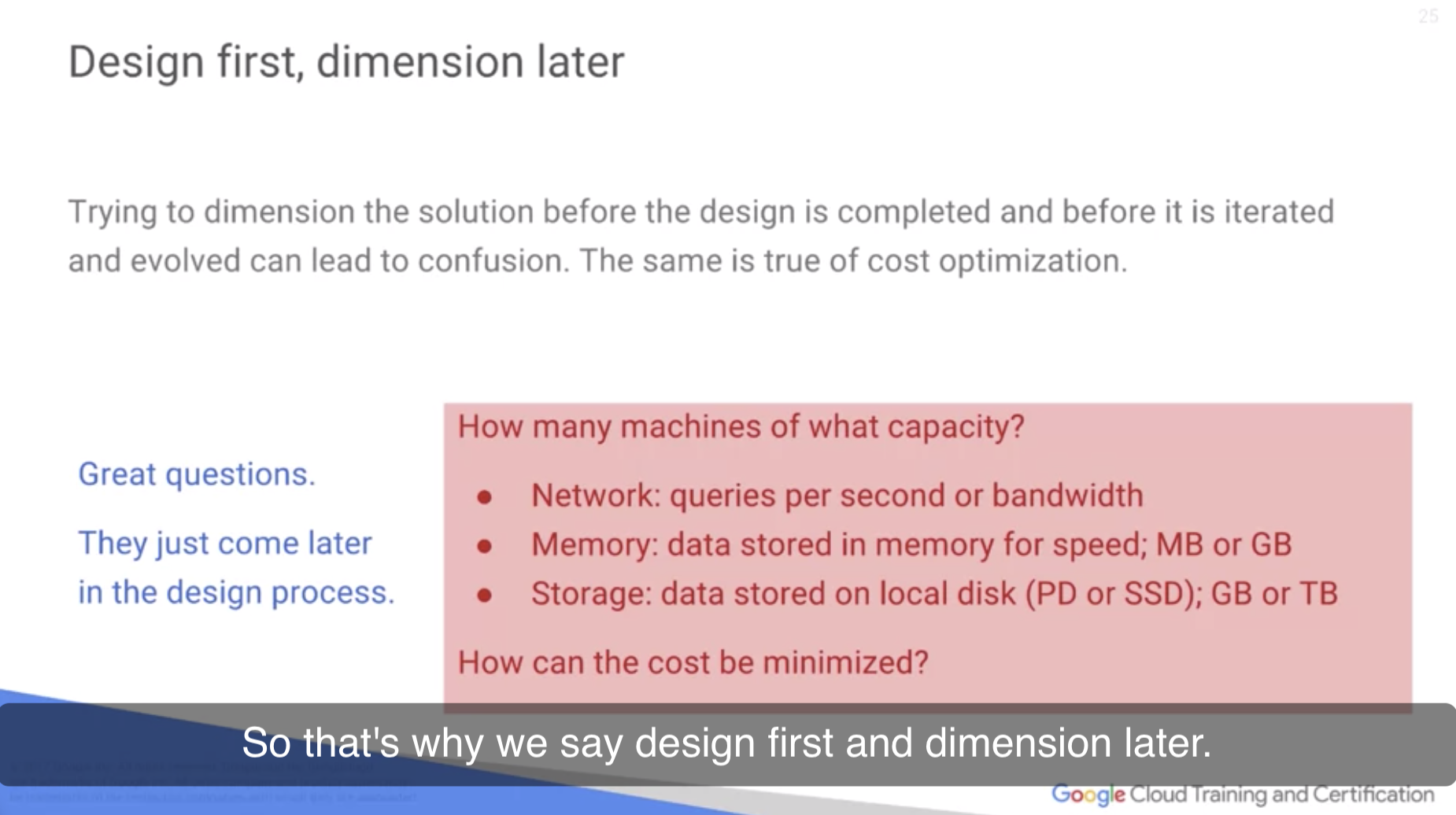
- 어떤 종류의 메모리가 필요한가? High-speed?
- disk는 어떤 걸 써야 하는가?
등등의 문제는, 나중에 바꿔도 된다. 그게 Cloud의 장점이니까.
그렇지만, 이걸 미리 고민하고 설계하는 건 scale할 때 매우 중요해진다. 나중에 tune / optimize할 때 유용하기 때문.